Generating Images using Genetic ProgrammingΒΆ
Creamas offers some ready made objects to start producing images using genetic programming (GP), which can be found from
gp
package. They are coupled with image evaluation features from
features
module. The functionality is based on
DEAP library.
Below is a simple example of creating images using GPImageGenerator
.
from creamas.domains.image.features import ImageEntropyFeature
from creamas.domains.image.gp import tools
from creamas.domains.image import gp
# Create a primitive set for DEAP.
pset = tools.create_super_pset(bw=True)
# Create a DEAP toolbox with some reasonable default values for quick testing.
toolbox = tools.create_toolbox(pset)
# Choose a feature which should be maximised and create an evaluation function for it
# (Remember that evaluation function should also return possible framing for the artifact.
feat = ImageEntropyFeature()
def evaluate_func(artifact):
# Filter out uninteresting images
if gp.GPImageArtifact.png_compression_ratio(artifact) <= 0.08:
return 0.0, None
return feat(artifact), None
# Create a GP image generator, which adds 'name' as created artifacts' creators.
# If each agent has its own generator, 'name' should be different for each agent.
# Using e.g. util.sanitize_agent_name(agent.name) is a good choice.
gpgen = gp.GPImageGenerator('name', toolbox, pset, 40, 20, evaluate_func, (32, 32))
# Run evolution with given parameters and return the best artifact
arts = gpgen.generate()
art = arts[0][0]
img = art.obj
# Save the artifact as an image and the function used to generate it. Functions can be used again to generate the
# image from same function in different sizes, or to induce succesive GP runs.
gp.GPImageArtifact.save(art, 'test_image.png', pset, shape=(400, 400), string_file='test_image.txt')
For example, the code above has been used to generate the following image:
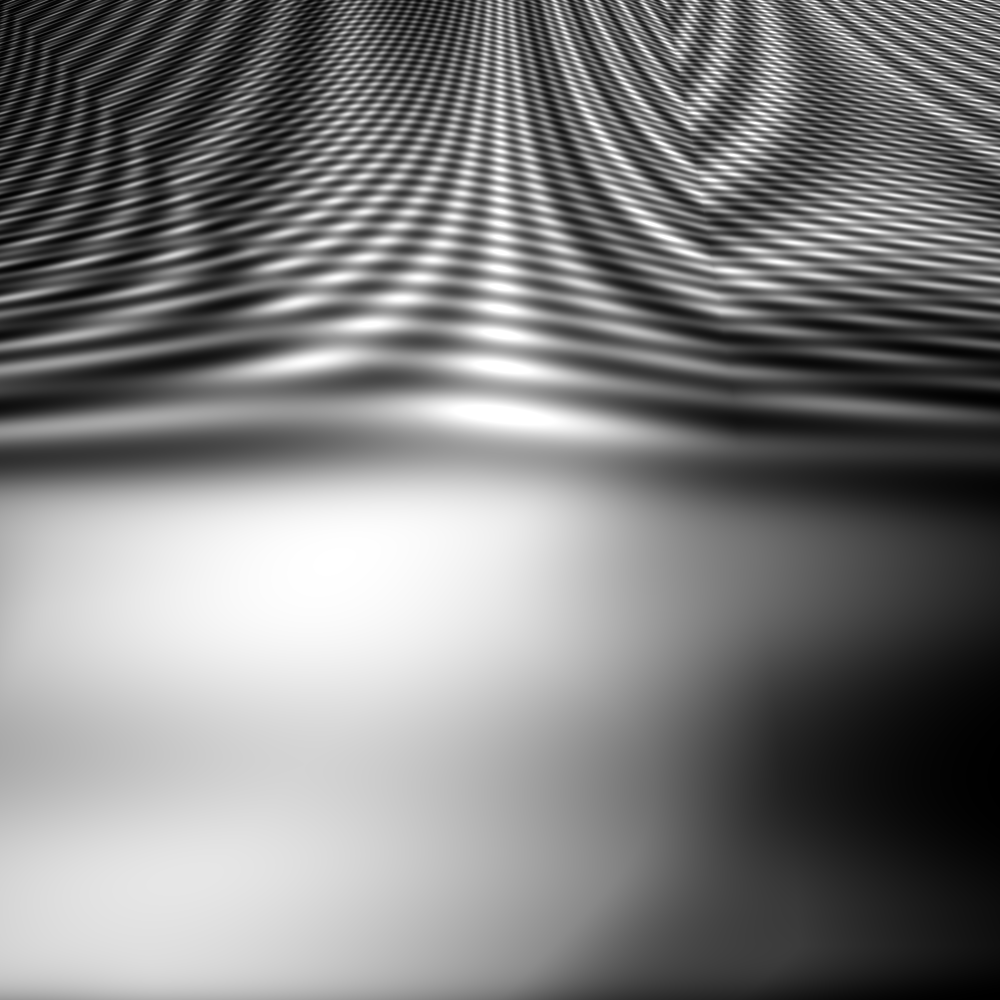
Check out GP documentation for details on how to use the functionality.